Posted by coljac in Astrophysics | 0 Comments
Deeper Wider Faster
The last couple of days I’ve been helping out – basically, as semi-skilled labour – on an exciting astronomy collaboration happening here in Melbourne and around the world. In astronomical terms, a million years is as the blink of an eye. When you are studying the expansion of the universe, the evolution of galaxies and the lifetimes of most stars, one is often talking in gigayears – time scales of billions of years.
However, there are astronomical phenomena that occur much more rapidly. Variable stars pulsate in hours and days; pulsars spin in seconds or less, ticking away over the aeons; supernovae explode in minutes and fade away in weeks and months. There may be other objects and phenomena of interest to science that operate on even shorter timescales. The problem is: If you aren’t sitting there, constantly watching the sky, how would you ever catch one?
The Deeper, Wider, Faster collaboration is designed to do just that and catch some of these fast-happening cosmic events. Astronomers in observatories around the world – Australia (including Jeff Cooke here at Swinburne and my fellow PhD students Igor Andreoni and Dany Vohl), the USA, Chile, and even Antarctica and in space – are all monitoring the same patch of sky, taking rapid exposures of a few minutes each of large fields using different parts of the electromagnetic spectrum. In order to catch transient (i.e. fast and temporary) events, a pipeline of software compares the new images with a template to see if anything has changed. By subtracting two images from one another, bits that remained the same should cancel out to nothing, but anything that has changed – whether brighter or dimmer – should stand out. These brighter or dimmer patches are then filtered to catch the most interesting ones, but ultimately need to be looked at by a human being to determine whether they are an artefact of the process (say, a bad pixel in the camera) or a real event somewhere in the universe.
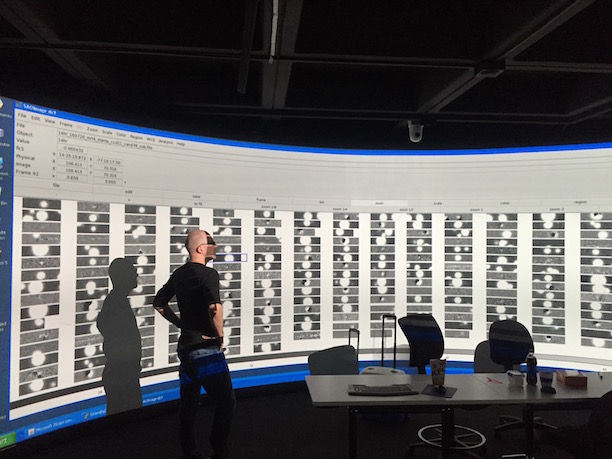
Me, pretending to look at the data.
The data the humans have to look at is complex in its simplicity. Ultimately, the object that will be found will be bright grey blobs in any single image. The problem is that artefacts caused by, say, a misaligned image subtraction or a ghost image in the electronics of the detector might also look like little blobs. Spotting the difference can be subtle and requires a bit of practice. Here’s a bad subtraction – some parts too bright, some too dim:
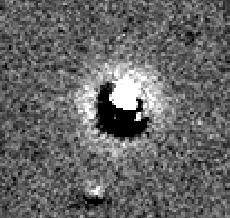
Bad subtraction.
The middle of these two, on the other hand, just might be something:
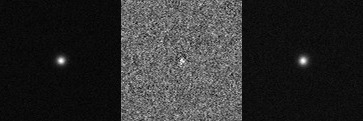
A candidate transient. The left image is the “before” photo, the right, after . The difference between the two is in the middle. Has it changed brightness?
Since the cameras are snapping pictures every few minutes, there’s more data always coming in to help verify candidates. In the case of a real transient, you’d expect the brightness to vary from image to image – either rising and falling or appearing and disappearing for the fastest of events. From this change in brightness over time, you can generate a light curve like the one pictured below for a closely-observed supernova:
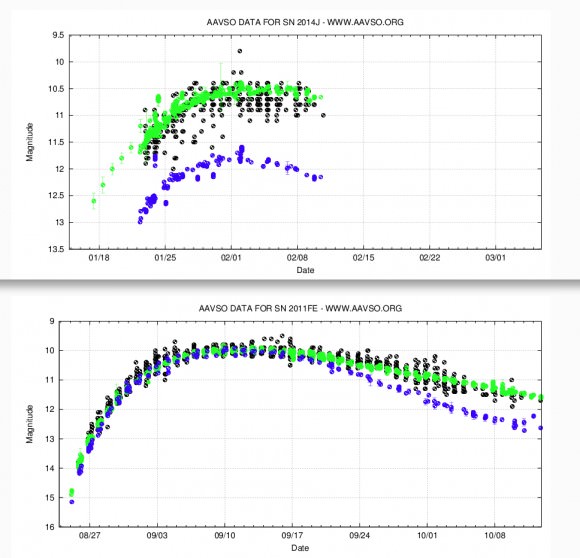
Supernova light curve. Source: Universe Today/American Association of Variable Star Observers
Even with only 5 minutes between observations you can calculate how bright something is and make such a curve. Here’s one that popped up in real time in the control room:
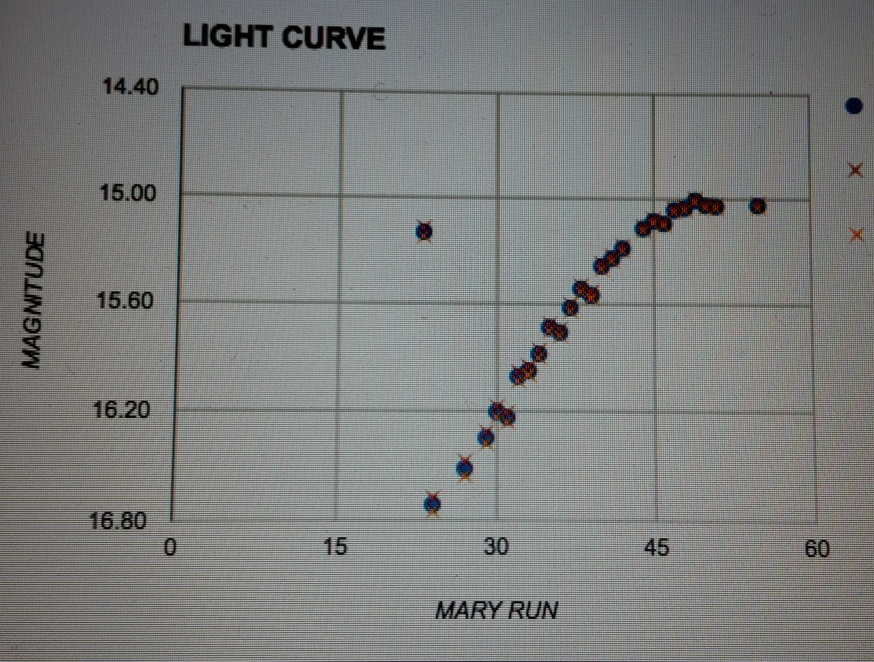
A light curve of an object discovered in real time. Photo courtesy Igor Andreoni
Interesting – there is definitely something changing there!
Between the smooth blob picture and a rising or falling light curve a human can pretty quickly sort the wheat from the chaff, and a trained amateur can do after minutes or hours of training. So, what about learning machines? Although there is considerable sophistication here already in filtering out the junk so humans just look at the most promising candidates, there is clearly room for some deep learning to be applied here at the final stage.
There are two problems with throwing a neural network at this challenge. Firstly, a training set of good and bad candidates needs to be assembled so the computer has something to learn from. Secondly, one of the aims of Deeper, Wider, Faster is to find events that have never been observed before. So how could an algorithm learn to find things novel to science?
Like all things, the best answer is probably a mixture. For instance, I’m now sorely tempted to explore the issue of bad subtractions and see whether my artificial neural networks can get a handle on those. If that worked, then the humans would have more time to spend examining more promising candidates. At any rate, the project continues over the next week or so – let’s hope they find something novel and bizarre!